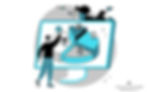
Market research has evolved into an intricate tapestry of data analysis and consumer understanding. The quest for deeper consumer insights has birthed a technique of remarkable sophistication: cluster segmentation.
Advanced segmentation techniques have emerged as the golden key to unlocking the deepest layers of consumer behavior, preferences, and needs. While traditional segmentation relied heavily on demographics, advanced techniques transcend these surface-level characteristics.
What is Cluster Analysis and Segmentation?
Cluster segmentation is a sophisticated statistical technique that groups consumers into clusters based on their similarities in various dimensions.
In the realm of market research, cluster analysis is key as many customers may belong to a few segments, and customers are similar within a segment, but different across segments. Meaning it is imparative to analyze each segment separately.
Cluster analysis transcends the superficial confines of demographics, venturing into psychographics, behaviors, preferences, and more.
In this realm, consumers aren't mere data points; they become individuals united by shared characteristics, forming clusters akin to stars in a constellation.
Cluster analysis is diverse and can be used in a variety of applications ranging from consumer segmentation, identifying competitive sets of products, or groups of assets whose prices co-move, or even geo-demographic segmentation.
Cluster Analysis: A Stepwise Approach
Data Collection and Preparation
The journey begins with comprehensive data collection, including variables that capture diverse aspects of consumer behavior.
These variables could range from purchase history to online engagement patterns. The "similarities" and "differences" between data observations can be mathematically defined using distance metrics.
One key aspect of performing a cluster analysis is precisely defining the distance metrics, based on contextual knowledge and not solely mathematical knowledge.
While data can be clustered even if it is not metric, it is imperative to assign numerical meaning to data so that statistical analysis can be easily performed. The main reason is due to the fact that clustering relies on defining distances between observations, and more often than not, distances are defined solely with metric data.
Normalization and Standardization
Once data is prepared, variables are standardized to ensure uniformity and prevent one variable from dominating the analysis due to scale differences.
Having variables with difference ranges or scales can be problematic, as most of the results from clustering can be driven by a few large values.
To avoid this problem, it is important to standardize the data by making scaling of the initial raw attributes, for example having a mean of 0 and standard deviation of 1, or scaling them between 0 and 1.
Deciding which variable to use in a cluster analysis is a critically important decision which impacts the solution highly. Therefore careful selection is necessary.
Exploratory research provides a good sense of which variables can distinguish people, products, assets, or regions. This step is when contextual knowledge is highly relevant.
It is important to note that the data will be attributed into segmentation attributes and profiling attributes. The segmentation attributes will help organize the data, and the profiling attributes will help profile the data into clusters.
For example, within market research and segmentation, attitudinal data may be used for segmenting customers based on needs and attitudes towards a product or service, and then demographic and behavioral data will be used to profile the segment found.
Defining Similarity Measure
The goal of clustering and segmentation is to group observations based on how similar they are, therefore, it is crucial to have a good understanding of what makes two observations "similar".
If there is no good understanding of what makes two observations "similar", then no statistical method will be able to divulge an answer either.
Most statistical methods used in clustering and segmentation use common mathematical measures of distance, for example, Euclidean distance or Manhattan distance.
Choosing the Right Algorithm
Selecting the appropriate clustering algorithm is crucial. Each algorithm has its strengths, nuances, and applicability. K-means, Hierarchical Clustering, and Gaussian Mixture Models are some popular choices, each with its nuances and mathematical foundations.
Hierarchical Clustering is a method which helps visualize the data and how it is clustered together. It generates a plot called the Dendrogram which is helpful in visualization and helps indicate how the clustering method works, how observations are grouped together, starting with pairs of individual observations and merging smaller groups into larger ones, depending on which are closest to one another, like a tree branching.
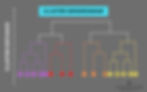
Dendrograms are a helpful visualization tool for segmentation, even if the number of observations is large, the tree typically grows logarithmically with the data.
However, dendrograms can be misleading, as once two data points are merged into the same segment, they remain in the same segment throughout the tree. This rigidity may indicate that dendrograms are useful in garnering an understanding of the data, especially in understanding the potential number of segments within the data.
K-means iteratively moves cluster centroids to minimize the sum of squared distances between data points and centroids.
K-means excels in creating spherical clusters and is computationally efficient. However, it's sensitive to initial centroid placement, leading to potential convergence at local optima. To mitigate this, multiple initializations and the 'elbow method' can be employed.
Gaussian Mixture Models (GMM) shine as a versatile and powerful technique that unveils the underlying structure within data.
GMM conceptualizes each cluster as a Gaussian distribution, characterized by its bell-shaped curve. However, unlike conventional clustering methods that assign rigid membership, GMM introduces a probabilistic approach.
Data points are viewed as harmonies that can blend across clusters, each with a probability indicating its affinity to various clusters.
Determine Optimal Clusters
One of the critical steps is deciding the optimal number of clusters. This could involve techniques like the Elbow Method, Silhouette Score, or Gap Statistic. Determining optimal clusters requires a keen eye for patterns and a systematic approach.
Overall, selecting the number of clusters requires a combination of statistical reasoning, judgement, interpretability of clusters, actionable value of clusters discovered, and many other quantitative and qualitative criteria.
All in all, different segments should be explored and the final choice should be made on statistical and qualitative criteria.
The Elbow Method provides graphical insights, where the x-axis represents the number of clusters and the y-axis denotes the within-cluster sum of squares (WCSS) – a measure of how spread out the data points are within a cluster.
As the number of clusters increases, the WCSS decreases, as clusters become more focused. However, at a certain point, adding more clusters leads to diminishing returns in terms of reducing WCSS.
The Elbow Method suggests that the 'elbow point' – the point where adding an extra cluster doesn't significantly reduce WCSS – is the optimal number of clusters.
The Silhouette Score measures how well each data point fits its assigned cluster compared to other clusters.
It ranges from -1 to 1, where higher values indicate better-defined clusters. A high Silhouette Score signifies that data points are well-matched within their clusters and far from neighboring clusters.
The Silhouette Score considers both cohesion within clusters and separation between clusters, offering a holistic perspective.
Gap Statistics compare the performance of your clustering solution against a random distribution of data points.
It assesses the gap between the within-cluster dispersion of your data and the dispersion you'd expect from random data. If your clustering solution has a significantly smaller gap, it suggests that the clusters formed are distinct and meaningful.
The Gap Statistics method calculates the gap statistic for different cluster numbers. The cluster number that yields the largest gap indicates the optimal number of clusters. Gap Statistics bring a statistical rigor that helps mitigate the subjective nature of other methods.
Cluster Assignment
Using the chosen algorithm, consumers are assigned to clusters based on their similarity scores across variables.
Cluster assignment isn't just about categorizing data; it's a window to understanding inherent relationships and patterns.
Cluster assignment transcends the realm of data points, transforming them into integral parts of a larger picture. Just as a jigsaw puzzle finds its complete image through the fitting of individual pieces, cluster assignment uncovers the intricate mosaic of data relationships.

The Impact: Unveiling Insights and Tailored Strategies
Cluster segmentation isn't just a technique; it's a portal to profound insights. By grouping consumers with similar behaviors and preferences, it unveils the intricacies of consumer segments that might have remained hidden.
This knowledge becomes the cornerstone of tailored marketing strategies, product offerings, and customer experiences.
The Pros:
Precision Marketing: Cluster segmentation eliminates the one-size-fits-all approach. Marketers can customize messages and offerings for each cluster, maximizing relevance.
Resource Optimization: Resources are channeled strategically toward segments most likely to respond positively, optimizing the return on investment.
Product Development: Insights from cluster segmentation guide product development, ensuring alignment with consumer needs and desires.
The Cons:
Complexity: Cluster analysis demands a solid understanding of statistics and algorithms, making it inaccessible to those without the necessary expertise.
Overlapping Boundaries: Real-world consumer behavior can be complex and multidimensional, leading to clusters with overlapping boundaries that are challenging to interpret.
Conclusion
In the grand tapestry of market research, cluster segmentation is the masterpiece that bridges the gap between data and insights. To the astute and highly educated minds, it offers a lens through which consumer behavior transforms into actionable intelligence. As we navigate this realm of complexity, impact, and potential drawbacks, we realize that beneath the surface of data lies a symphony of human behavior, waiting to be harmonized through the art of cluster segmentation.
If you are interested in learning more about our research tools and methods to help build your brand and deepen your marketing, check out our..
If you are interested in learning more about trends and insights in the luxury sector, check out our articles: